Research
I study coupled climate dynamics, including radiative feedbacks and climate sensitivity, climate variability, Earth's energy budget in the paleoclimate record, and atmosphere-ocean-ice interactions. I am currently investigating how climate feedbacks and Earth's energy budget have changed over the Last Glacial Maximum (~21 thousand years ago), Pliocene (~3 million years ago), and recent industrial era (1850-present). In this work, I am focusing on the role of spatial patterns of sea-surface temperature (SST) in determining radiative feedbacks, i.e., the "pattern effect."
Tools that I use: I run custom experiments in global climate models (using CESM), I use statistical methods (data assimilation and linear inverse models) to reconstruct past climate periods, and I incorporate observations (e.g., measurements of ocean temperatures and atmospheric pressure from ships of opportunity, ocean surface wave spectra from buoys and moorings, etc.) whenever possible. I enjoy research problems that require thinking in terms of probability.
SST Pattern Effect in the Paleoclimate Record
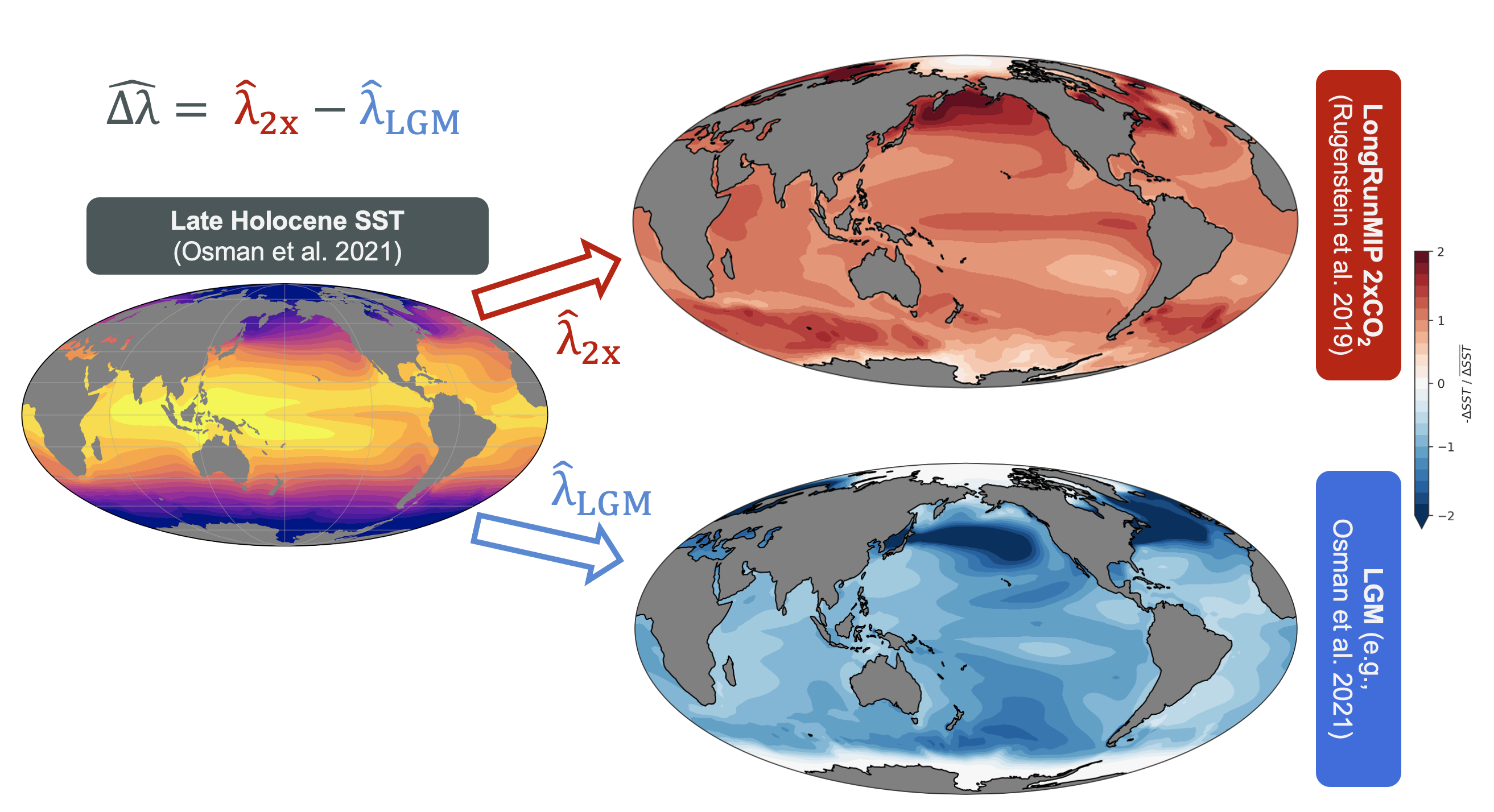
SST anomaly patterns from 2xCO2 compared to the Last Glacial Maximum
In atmosphere-only models with the LGM and 2xCO2 SST patterns prescribed as lower boundary conditions, we can estimate the climate feedback associated with each SST pattern. We repeat this in different atmosphere models and using different versions of LGM SST patterns, and we systematically find that the LGM feedback is less negative compared to the 2xCO2 feedback.
This work focuses on how the spatial pattern of sea-surface temperature (SST) impacts climate feedbacks and sensitivity (the "pattern effect"). We are using atmosphere-only GCMs to quantify differences in the radiative feedbacks actuated by LGM SST anomalies compared to the feedbacks we expect from a doubling of CO2. I have been running prescribed-SST experiments in NCAR's CAM4, CAM5, and CAM6 with reconstructions of SST and sea ice based on paleoclimate data assimilation, and I have worked with collaborators to run similar simulations in the UK Met Office model, HadGEM3, and NOAA's GFDL-AM4.
If we use the LGM as a constraint on the climate sensitivity that applies to modern warming (as done in Sherwood, Webb et al. 2020), we need to make an adjustment for how these different SST patterns (LGM vs. 2xCO2) drive different radiative feedbacks (and climate sensitivities).
For the Pliocene (a past warm period ~3 million years ago), we have found that smaller ice sheets and enhanced vegetation both influence the SST patterns over distant oceans, causing positive climate feedbacks from changes in cloud cover.
Carbon Brief invited an article explaining our findings on Climate Sensitivity based on the Last Glacial Maximum: Guest post: Ice-age analysis suggests worst-case global warming is less likely .
I also gave a 20-minute talk explaining this work at the ECS & Cloud Feedback Symposium (Oct. 2023): video recording.
For the general public, Phys.org, The National (UK), and UW News published brief articles that summarize our results.
Cooper, V. T., Armour, K. C., Hakim, G. J., Tierney, J. E., Osman, M. B., Proistosescu, C., Dong, Y., Burls, N. J., Andrews, T., Amrhein, D. E., Zhu, J., Dong, W., Ming, Y., and Chmielowiec, P. Last Glacial Maximum pattern effects reduce climate sensitivity estimates (2024). Science Advances. doi.org/10.1126/sciadv.adk9461
Climate Data Assimilation
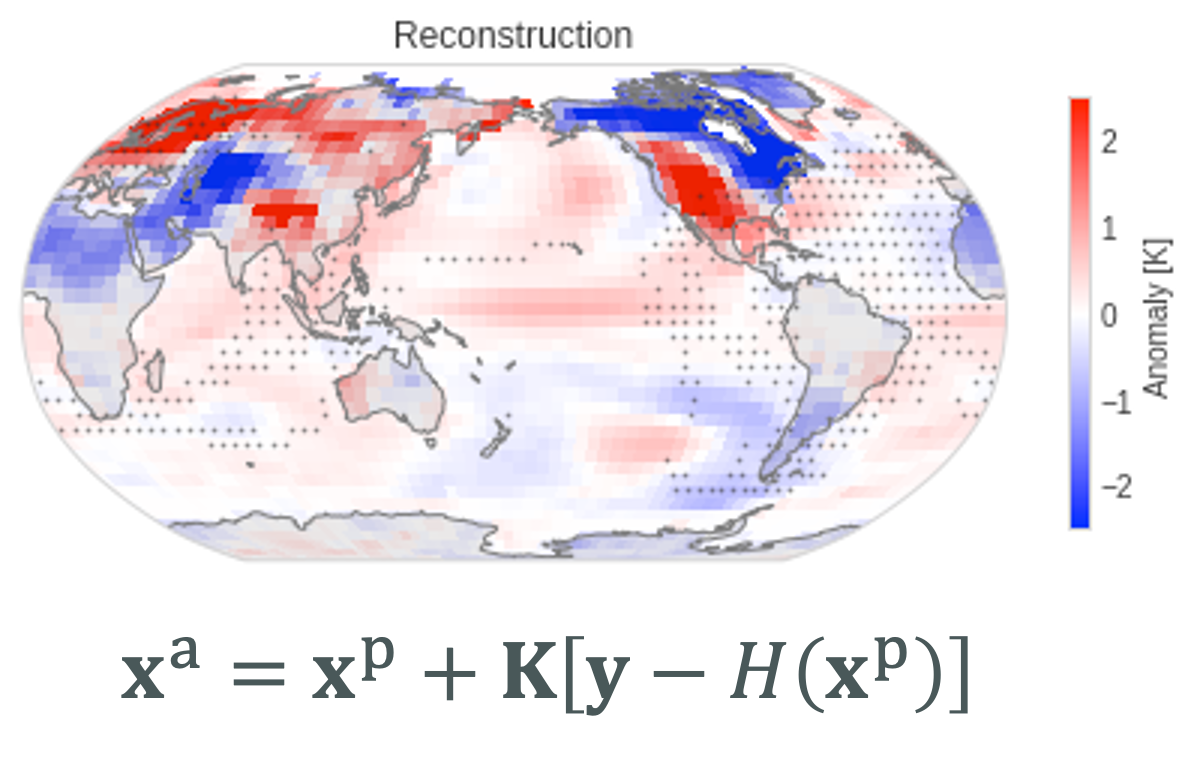
Reconstruction of global SST and land surface temperature in the 1850s.
Illustrative reconstruction of the global pattern of surface temperature informed by a small subset of observations (gray dots).
The observations are a representative example of the available SST data for reconstructing one month in the 1850s. This illustration uses an ensemble Kalman filter.
While we have reasonable constraints on the global-mean change in surface temperature back to 1850, we do not have strong constraints (nor robust uncertainty quantification) on the spatial pattern of SST change over the historical record. Past attempts at infilling gaps in SST observations back to 1850 have produced patterns of SST anomalies that differ substantially from each other, and those discrepancies in past warming patterns lead to large uncertainties in climate sensitivity inferred from the historical record. Improved estimates of SST patterns in the recent past could provide better information on climate variability and the climate system's response to anthropogenic forcing since the pre-industrial period.
Data assimilation enables reconstruction of full spatial fields of SST and sea ice back to 1850 at monthly temporal resolution. This framework is essentially Bayesian and propagates information from sparse observations (e.g., bucket measurements of SST from ships of opportunity) to unobserved regions of the globe. Data assimilation spreads this information using spatial covariances that are based on dynamics of the climate system (rather than interpolating without considering physical constraints). We are simultaneously able to quantify uncertainty in the spatial patterns.
Linear inverse models can be efficiently paired with data assimilation. After training a linear inverse model (LIM), we can use the LIM to predict SST patterns for a specific month based on our analysis of the previous month. The LIM predictions may improve our "prior" (i.e., first guess) estimate of the SST pattern, relative to the "uninformed prior" we would use in offline data assimilation, thereby enhancing our ability to constrain each month of SSTs back to 1850. The LIM is a computationally efficient statistical tool that captures the linear dynamics of a complex system.
Cooper, V., G. Hakim, and K. Armour.
Monthly Sea-Surface Temperature, Sea Ice, and Sea-Level Pressure over
1850–2023 from Coupled Data Assimilation.
Submitted, Journal of Climate.
Preprint: doi.org/10.31223/X5JH8K.
[PDF]
Polar Climate (M.S. Degree)
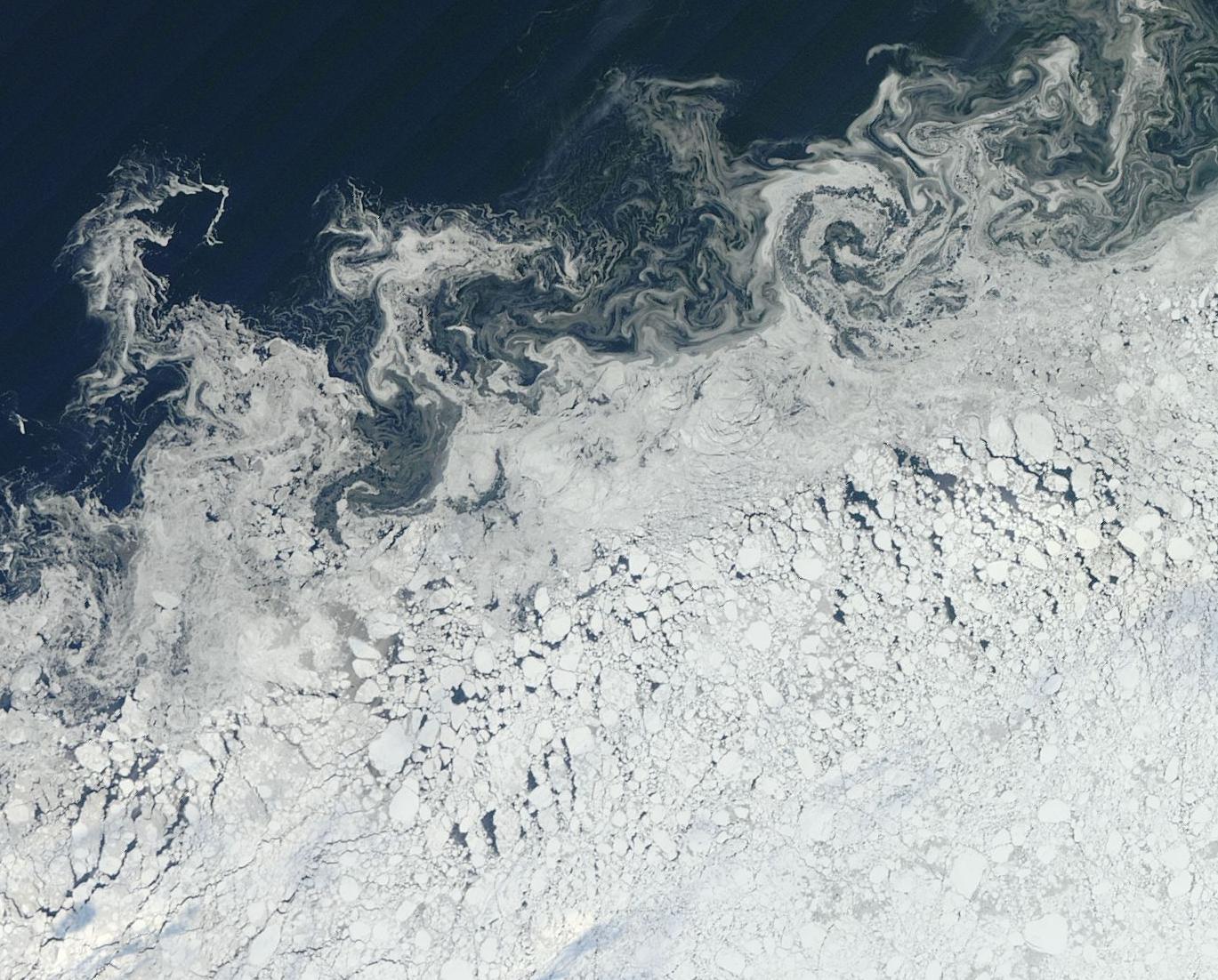
Marginal Ice Zone with Fragmented Ice Floes (Central Arctic, Oct. 2018)
Source: NASA EOSDIS Worldview.
Ocean surface waves affect sea ice near the ice edge, but waves can also develop in the smaller areas (width of ~10 km) of open water within the pack ice.
We've characterized those locally generated waves in observations and investigated how they could affect sea ice in a climate-scale coupled model.
With Arctic Sea Ice retreating due to global warming and Arctic amplification, there are large regions of the Arctic Ocean which are now ice-free during at least part of the year. It is well established that ocean surface waves can develop in these open water regions and propagate into the pack ice. Waves fracture ice into smaller "ice floes" and can cause a positive feedback during the summer alongside the ice-albedo feedback.
At distances of more than 100 km inside the large-scale sea ice edge, measurements showed locally generated wind waves during the Arctic summer. We found that these waves develop in pockets of open water within the pack ice.
I've conducted a series of experiments in a global coupled wave-ice model (using the CESM framework and building on Roach et al. 2019). These experiments attempt to reproduce the local wind waves in partial sea ice cover and explore how uncertainties in wave-ice physics affect polar oceans in global, climate-scale simulations.
See a poster and paper from my M.S. thesis on this topic below.
[Download Polar AMS 2021 Poster].
Cooper, V. T., Roach, L. A., Thomson, J., Brenner, S. D., Smith, M. M., Meylan, M. H., and C. M. Bitz. Wind waves in sea ice of the western Arctic and a global coupled wave-ice model (2022). Philosophical Transactions of the Royal Society A. 380:20210258. https://doi.org/10.1098/rsta.2021.0258
Collaborators: Lettie Roach, Cecilia Bitz, Jim Thomson, Sam Brenner, Maddie Smith, and Michael Meylan.